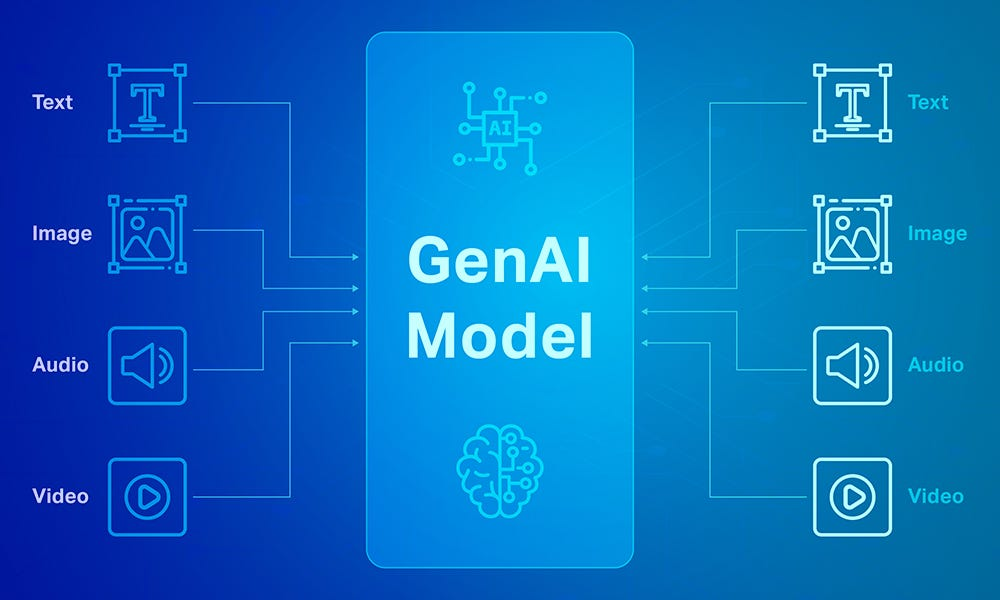
In the vast ocean of data that surrounds us, information rarely exists in isolation. We encounter data in various forms – text descriptions accompanying images, audio narrations explaining videos, sensor readings paired with location data, and much more. Traditional machine learning models often focus on analysing single data modalities in isolation. However, a powerful and increasingly relevant approach, known as multimodal learning, aims to bridge this gap by enabling models to understand and reason across multiple data types. This capability unlocks a wealth of potential for solving complex real-world problems, making it a crucial area of study for anyone considering a Data Scientist Course.
The Power of Synergy: Why Multimodal Learning Matters
Our own human intelligence thrives on integrating information from different senses. We understand a situation better when we see a facial expression, hear the tone of voice, and read the accompanying text. Multimodal learning in data science seeks to replicate this ability in artificial intelligence, allowing models to gain a more comprehensive understanding of the world. By fusing information from different modalities, such as text, images, audio, video, and sensor data, we can often achieve significantly better performance and gain deeper insights compared to analysing each modality in isolation. This synergy is what makes multimodal learning such a promising frontier in the field.
Real-World Applications: Multimodal Learning in Action
The practical applications of multimodal learning are rapidly expanding across various industries. Here are some compelling use cases that highlight its power:
1. Enhanced Sentiment Analysis
Traditional sentiment analysis often relies solely on text data. However, human emotion is conveyed through more than just words. Multimodal sentiment analysis combines text with audio cues (like tone and pitch) and visual cues (like facial expressions) to provide a richer and more accurate understanding of sentiment. For instance, a customer review might contain positive words but be delivered in a sarcastic tone. A multimodal model can capture this nuance, leading to more accurate sentiment classification. This is a valuable skill for those completing a Data Scientist Course in Pune, as businesses increasingly seek to understand customer emotions holistically.
2. Improved Image and Video Understanding
Analysing images and videos becomes significantly more powerful when combined with textual descriptions or audio narrations. For example, in image captioning, a model learns to generate textual descriptions of images by jointly analysing visual features and language patterns. Similarly, in video understanding, combining visual information with audio and subtitles allows models to understand events, identify objects, and interpret actions more effectively. This has applications in areas like autonomous driving (understanding the surrounding environment through cameras, lidar, and potentially audio cues) and content analysis (automatically tagging and categorising videos based on their visual and auditory content).
3. More Accurate Healthcare Diagnostics
In healthcare, multimodal learning can integrate various data types like medical images (X-rays, MRIs), patient history (textual records), and physiological signals (ECG, EEG). By analysing these diverse data streams together, AI models can potentially provide more accurate and timely diagnoses, predict disease progression, and personalise treatment plans. For example, combining image analysis with patient records could help identify subtle patterns indicative of a disease that might be missed by analysing each modality separately.
4. Personalised Education and E-learning
Multimodal learning can revolutionise education by creating more engaging and personalised learning experiences. By analysing a student’s interaction with learning materials (e.g., text read, videos watched, audio responses), their facial expressions (indicating engagement or confusion), and their written responses, AI systems can gain a deeper understanding of their learning style and progress. This information can then be used to tailor the learning content, provide personalised feedback, and identify areas where a student might be struggling.
5. Advanced Human-Computer Interaction
Creating more natural and intuitive human-computer interfaces is another promising area for multimodal learning. By combining speech recognition, facial expression analysis, and gesture recognition, AI systems can better understand user intent and respond in a more context-aware manner. This is crucial for developing more sophisticated virtual assistants, robots that can interact naturally with humans, and more accessible technologies for individuals with disabilities.
6. Enhanced Fraud Detection
Financial fraud often involves a combination of suspicious activities across different channels, such as unusual transaction patterns (numerical data), inconsistent account information (textual data), and potentially even voice anomalies during phone interactions (audio data). By analysing these multimodal signals together, fraud detection systems can identify fraudulent activities with greater accuracy and reduce false positives. The ability to build such sophisticated detection systems is a valuable asset for graduates of a Data Scientist Course.
7. Context-Aware Recommendation Systems
Traditional recommendation systems often rely on a single data modality, such as past purchase history or user ratings. Multimodal recommendation systems can significantly enhance the accuracy and relevance of recommendations by incorporating other modalities like product descriptions (text), images, user reviews (text and potentially sentiment), and even audio/video demonstrations of the product. By understanding the user’s preferences and the product’s characteristics across multiple dimensions, these systems can provide more personalised and satisfying recommendations.
Building Multimodal Models: Challenges and Approaches
Developing effective multimodal learning models presents several challenges, including:
- Data Heterogeneity: Different modalities have different statistical properties and representations.
- Information Fusion: Effectively combining information from different modalities is a complex task.
- Temporal Alignment: When dealing with sequential data like video and audio, aligning information across time is crucial.
- Interpretability: Understanding how a multimodal model makes decisions can be challenging.
Various techniques are employed to address these challenges, including:
- Feature Extraction: Extracting meaningful features from each modality using modality-specific models (e.g., CNNs for images, RNNs for text).
- Feature Fusion: Combining the extracted features using techniques like concatenation, element-wise operations, or attention mechanisms.
- Joint Representation Learning: Learning a shared representation space where information from different modalities can be effectively compared and combined.
- Cross-Modal Attention: Allowing the model to focus on the most relevant information from different modalities when making predictions.
The Future of Multimodal Learning
As data continues to grow in complexity and variety, multimodal learning will undoubtedly play an increasingly significant role in data science. Advancements in deep learning and access to more diverse datasets are driving innovation in this field. We can expect to see even more sophisticated multimodal models emerging, capable of tackling increasingly complex real-world problems and providing deeper, more nuanced insights. For those embarking on a Data Scientist Course in Pune or elsewhere, mastering the principles and techniques of multimodal learning will be a key differentiator in the years to come.
Conclusion: Embracing the Richness of Multimodal Data
Multimodal learning represents a powerful paradigm shift in data science, moving beyond the limitations of single-modality analysis to unlock the rich information contained in the diverse forms of data that surround us. By enabling machines to understand and reason across text, audio, images, video, and other data types, we can create more intelligent, robust, and human-like AI systems with a wide range of practical applications across various industries. As the field continues to evolve, the ability to effectively leverage multimodal data will be a defining characteristic of cutting-edge data science and a valuable skill for aspiring and practising data scientists alike.
Business Name: ExcelR – Data Science, Data Analytics Course Training in Pune
Address: 101 A ,1st Floor, Siddh Icon, Baner Rd, opposite Lane To Royal Enfield Showroom, beside Asian Box Restaurant, Baner, Pune, Maharashtra 411045
Phone Number: 098809 13504
Email Id: [email protected]